Financial companies are quickly amassing vast volumes of data, but executives are increasingly challenged by the fractured nature of existing systems
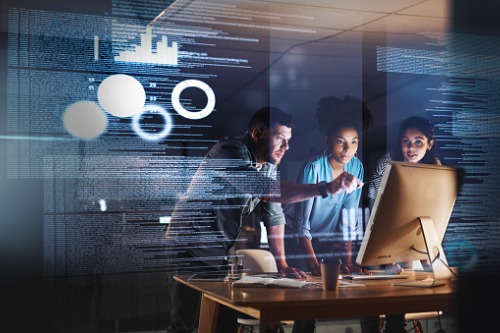
Almost all financial services executives are challenged by high volumes of data, but continue to ramp up their data collection despite it being difficult to put that data to its highest and best use.
Banks and financial institutions have had access to data and analytics surrounding that data for decades. However, with the growing amounts of data available—mobile data, clickstream data, voice data, call centre data—new opportunities abound in the marketplace.
Matt Fabian, is the director of financial services research and consulting for TransUnion Canada, and said that today, financial executives are asking what learnings and insights can be gained from additional data that they didn’t have before or didn’t know what to do with before. More importantly, do those new insights help frame a better customer experience, better product design, or better service? Can operational costs be saved?
The integration of data is almost table stakes these days, Fabian added. The Amazon effect has taken hold on every aspect of consumer behaviour, and everyone expects mobility, agility, and easy accessibility when it comes to accessing their own information.
“To enable that, you need to make faster decisions better,” Fabian said. It's just the nature of how banking, insurance, and other financial services are evolving, and understanding data points has become critical in understanding the customer experience.
In October, TransUnion commissioned a study exploring the challenges faced by financial services and insurance industries. Canadian executives have a mixed outlook on how they're implementing AI and machine learning into their models and analytical solutions. On one hand, Canadian execs feel that they’re in a pretty good position to capitalize on increased use of artificial intelligence (AI). On the other hand, the majority of those surveyed don’t feel as if they can handle the new data.
Financial institutions have been focused on investing and incubating advanced analytics for a while now, often through their own innovation-type labs. Despite the numerous tools and their disposal that can perform machine learning and complex analytics. The challenge, Fabian says, is the data.
“You can have the best modeling software, the best cognitive neural networking algorithms in the world, and if you don’t have either the volume or the type of data for the models to be able to take advantage of it, you become a little bit more limited,” he said.
Where executives are really expressing concern is their ability to integrate the data. Many banks utilize decades-old limited legacy systems that are on longer, more predictive cycles. Even though institutions are now obtaining vast amounts of data at breakneck speed, this fresh data can’t necessarily integrate into their older systems and companies aren’t necessarily able to respond quickly.
New fintech companies are emerging that promise to help companies with this very problem. But when you’re steering a big ship, Fabian said, it’s that much harder to turn it around, and in this case, it’s also much more expensive.
“It’s a massive investment. It’s tens to hundreds of millions of dollars for a national bank to flip around all their old legacy systems, and at the same time, you can’t just turn the old ones off because the lights have to stay on,” Fabian said. “They’re all working away at it. It’s going to take a while. Some are farther ahead than others.”
Financial institutions are also susceptible for mergers and acquisitions over time, which compounds the issue because some systems get integrated with each other and others remain because they’re working well for their individual purpose. That is changing, however, as more formalized roles and teams around data analytics, strategy, and governance become commonplace. Canadian executives are understanding this, as the Aite Group study revealed that they’re constantly trying to work toward a long-term strategy regarding data as opposed to upgrading and managing tools bought for specific uses.
It's not only fintech companies that are moving in to capitalize on the stoic nature of banks and lending institutions; alternative lenders are starting to fill voids, Fabian said. Being smaller and perhaps nimbler than traditional lenders, these companies are determining whether more data can help them be smarter regarding clients that the banks have ignored.
Fabian calls this segment of the industry ‘lendtech’: those who have technology and AI but are focused on lending and/or issuing credit. This is different from the fintechs that are more focused on operations; instead of competing with the banks, they want to form partnerships and use their own AI or machine learning algorithms to optimize the lender’s mortgage origination process.
From a lender perspective, the focus is on operations and becoming faster and nimbler in order to take advantage of increased amounts of data and create a better customer experience.
More data is always better, Fabian said, and open banking isn’t far off. This could create a lot of opportunities for brokers.
“It opens up the data sources and opens up [interfaces] to allow open access to all sorts of lender data,” Fabian said. “If you’re a broker that can partner with an AI company or build an AI company . . . all of a sudden you’re going to have access to unprecedented amounts of data potentially, and how does that change your business?”
Companies aren’t necessarily waiting for open banking to be formalized; they’re starting to get access to alternative data sources now. Brokers who are able to access that intelligence will be better armed to go out on behalf of their clients and get the best deals.
“It might not be formally regulated process but we’re going to start to see more and more of that come, and I think that’s something that anybody in the mortgage space is going to look at,” Fabian said.