It's all about the data…
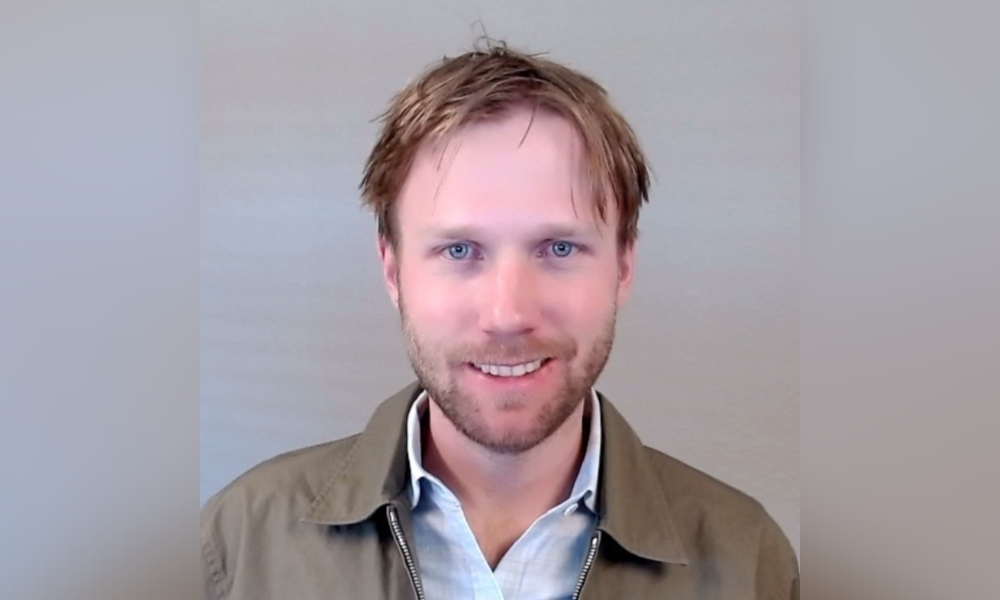
While some may view community redlining – the practice of actually circling in red ink low-income swaths of cities where lenders once were averse to expand borrowing – as an anachronism, the practice still exists to a lesser degree. Real estate data provider LightBox can zero in on such underserved areas as well as identify other areas of inequity, an officials told Mortgage Professional America.
A quick history lesson for context: Some 80 years ago, the Home Owners’ Loan Corp. a federal agency, created so-called “Residential Security” maps of major American cities. As the National Community Reinvestment Coalition (NCRC) explains on its website, the maps document how loan officers, appraisers and real estate professionals evaluated mortgage lending risk during the era immediately prior to the suburbanization surge of the 1950s. Neighborhoods considered high risk or “hazardous” were often redlined by banks, NCRC explains, denying them access to capital investment that had the potential to improve the housing and economic opportunities of residents.
Most of the areas deemed hazardous were populated by minorities at a time when segregation was the order of the day. Fast forward to the 21st century, and LightBox has overlaid its data set over those same maps to determine lending patterns that may point to persistent undercapitalization.
“What’s interesting about intersecting it with our data is we have the boundaries of every property in the country,” Zach Wade (pictured), vice president, Data Science, at LightBox, told MPA in a recent telephone interview. “So, by intersecting the publicly available data set with our structural level data set – which also includes things like home prices, what the building is, how big it is – we’ve been able to go in and look at a time period of transactions to see if there’s a persistent difference in areas that were historically redlined either by that loan corporation.”
Read more: CRE data provider LightBox acquires zoning advisory firm
While illegal redlining is now not overt, Wade noted, data comparisons can help detect remnants of unsavory lending practices of the past. “What we did is look at, given historical redlining that was made illegal, if there were still artifacts of it,” he explained. “That particular home loan corporation [Home Owners’ Loan Corp.] was pretty explicit of what was desirable versus undesirable, and race played an active role in their definition of it.”
The aim of overlaying new data with old maps is to detect any lingering underinvestment or economic stagnation in certain areas once redlined, he explained. “We didn’t actually do too much of a detailed analysis or take an opinion as much as create this data set others could look at to draw their own conclusions from.”
The precision of such granular data also can detect areas most prone to flooding, or parts of the country where there is a lack of broadband – other potential signs of inequity.
“We think a lot about exposure of populations to climate and if there’s a discrepancy with flood exposure in more generally disadvantaged communities,” Wade said. “What’s cool about our data is you can think about any general topic and, as long as there’s another data set out there we can merge into, we can do a structural level analysis.”
Read next: Bank of America expands minority lending capabilities
A more recent – and decidedly more noble – example of government area analysis came at the peak of COVID-19, when the government heightened efforts to provide internet service to areas of the country that were not connected – particularly rural or low-income sectors. Part of the impetus for the movement was the prevalence of schools shifting to virtual learning as residents adhered to the tactics of physical distancing to ward off the spread of illness.
The problem that emerged was that the government’s maps were representative of overly large areas or lacked structural data, making it difficult to determine where to allocate funding for fiber installation, Wade explained. “That’s really where our data is super important,” he said, “bringing it down to the structural level. It’s hard to determine where to allocate money at the structural level to make sure they deployed to the areas that actually needed it.”
It’s been said that those who don’t remember the past are condemned to repeat it. Today’s sophisticated data collection, when superimposed with mapping of the past, can yield a powerful memory aid to prevent injustices from occurring again.