Predictive analytics is everywhere. What can it do for the mortgage industry?
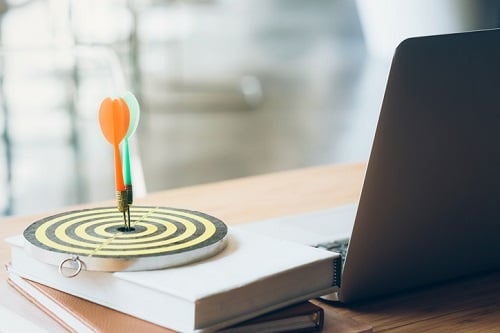
Predictive analytics is really just a fancy way of looking at existing data, establishing a pattern and then using that pattern to predict actions.
An easy way to think about predictive analytics is by logging into and viewing the home page on Amazon.com. Scroll down, where there is a list of items under the heading “Customers who bought similar items also bought . . .” Based on the items already purchased under a particular account, Amazon knows what else the buyer is likely to need and/or want.
There are two types of methodologies, statistics-based and neural networks-based that have been developed over the past several decades.
When it comes to the mortgage industry, predictive analytics is also being used to predict borrower behavior, the same as it is in any other industry. Gagan Sharma, president and CEO of BSI Financial Services said that predictive analytics is most likely to be used for performance characteristics, determining whether a borrower is going to default on a loan, or for determine whether or not a borrower is likely to prepay their loan.
“To our clients, a loan that pays off is not a good thing. If you are a mortgage banker and you own the mortgage asset, if the loan pays off, that’s a financial asset that walked out the door. So what we are looking at is user analytics for either doing a better job of identifying loans at risk of payoff, or looking at loans that may have a risk of falling behind.”
It’s not as simple as looking into the records of one individual database, or even within a single lender to see loan patterns. And, as Gagan points out, the data that does exist can be quite misleading. A borrower with a mortgage at 3.75% may have a higher likelihood of payoff than a borrower with a 5% rate, depending on other factors. The more data that exists, the more mortgage professions can see those other factors, and the more accurate those predictions are.
Bigger isn’t always better—except when it comes to data. Where does that leave the small mortgage teams without the same access to that data? How will they be able to bridge the gap as companies become even more data-driven when it comes to strategies behind client retention and gaining market share? When talking about the Davids and the Goliaths of the mortgage industry, it’s clear that data belongs to those massive companies, the loanDepots, the Quickens, who have the sheer volume of data and the technology to harness it.
“For the larger companies, they’ll be able to gain from that, because they just have to be able to be more efficient, be able to mine the data better, they’ll be able to close those few extra points of loans, or reduce the costs by a little bit extra, and truly, if you think about it, what is the difference between Amazon and me setting up a little website to sell things online? At the end of the day, all I’m doing is taking a product, putting it in a box, taking it to a UPS store and shipping it to somebody. But Amazon has so much data that they’re able to identify what I’m likely going to need and price it slightly better, and make me buy two extra things and that’s how they make so much more money than a little store down the street,” Gagan said. “I actually think that this technology thing makes it harder for smaller companies who are not tech savvy, it’s become the challenge of the future . . . if you don’t have that, then how do you survive in the future?”
Through the use of cloud technologies, computing power has become relatively inexpensive, and because of that, financial firms are now able to apply advanced computer science—probability, mathematical, and machine learning calculations—to the massive amounts of data at their disposal.
On one hand, it can help originators focus more closely on their targets.
“Once the lenders/originators know that a borrower is likely to payoff, they would need to adjust their marketing and borrower outreach processes targeting those borrowers. It may involve more frequent outreach, or creating special offers for those borrowers,” Sharma said, noting that BSI is currently doing this for some investor clients. “Our loan officers target those borrowers for outreach such that they consider us for their next loan, allowing our investor client to retain that borrower relationship and the new loan on their books.”
On the other hand, it could be used to act against a borrower’s best interest. Many borrowers don’t want another loan; paying off their mortgage is the end goal, full stop. Once a tool is available, though, it’s up to the user to decide what to do with it, and the most responsible originators would use predictive analysis through the lens of the borrower’s overall financial picture, not just as a way to keep them trapped in the system.
“In business, we as businesspeople get access to certain tools,” Sharma said. “The first aspect is, do you have access to the tools. The second aspect is, how do you actually use the tools, and not everybody knows how to use the tools with the same level of effectiveness, is something to think about.”
How predictive analysis will come to be used by originators remains to be seen, but implications could be huge.